Avoid These 3 Predictive Maintenance Pitfalls to Ensure Smart Building Success
Key Takeaways:
- Bad data corrupts AI-driven PdM: Inaccurate or incomplete data leads to false predictions and "AI hallucinations," resulting in unreliable maintenance decisions.
- Low-quality tech hinders performance: Cheap IoT sensors and poorly integrated software reduce data fidelity, leading to PdM failures.
- Cybersecurity is critical: OT and IoT system breaches can disrupt data integrity and predictive maintenance capabilities, making strong cybersecurity a PdM essential.
When we enter a modern smart building, we expect the lights to adjust appropriately based on ambient light levels, the temperature and humidity to be ideal, and a multitude of IoT and OT devices to work together seamlessly, providing an optimal experience for occupants. In this scenario, the success of smart building technologies largely depends on a robust and highly accurate predictive maintenance (PdM) framework to keep everything running smoothly.
Expectations are often far from reality, however. Despite all the advancements in smart building technologies, pitfalls do occur with PdM processes that can bring building technologies to a halt. Let’s examine a few common predictive maintenance pitfalls and how to avoid them, ensuring that your smart building operates as expected every time.
Pitfall 1: Bad Data
Despite all the advancements in artificial intelligence, it’s just that—artificial. It’s important to note that AI consistently produces accurate outcomes only when the data it analyzes and uses to make decisions is of high quality.
Inaccurate, incomplete, or redundant data sourced from smart building systems can result in false positives or negatives, leading to what are known as AI hallucinations. This happens when AI makes recommendations it thinks are correct but are, in reality, incorrect due to the poor-quality information it had to work with.
While we desire to be able to simply implement AI and allow it to take over smart building operations, this is not yet feasible. To get yourself out of a “bad data” pitfall, regular maintenance and data calibration checks are essential for ensuring that accurate and well-curated data is analyzed, preventing the automated, AI-driven predictive maintenance results from being skewed inaccurately.
Pitfall 2: Poor Quality Tech
According to Precedence Research, the smart building technology market has experienced a consistent 10% CAGR over the past decade, reaching over $100 billion today, with the same momentum expected to continue through 2034. This growth has attracted numerous new and low-cost entrants to the market, which may appear appealing in terms of return on investment at first glance but come with low-quality technology. This is especially true when it comes to building technologies that leverage IoT sensors and poorly coded software that’s not fully baked.
The result? Inaccurate or insufficient data inputted into predictive maintenance platforms, leading to erroneous predictions and resulting in incorrect maintenance recommendations.
If you've invested in subpar building technology and are left with a solution that falls short of your expectations, there are still opportunities to gain value from your investment. Here are some practical tips:
- Tune solution to vendor recommendations. Some smart building predictive maintenance solutions can perform well but require extra care when it comes to setup and calibration. Be sure to have your struggling systems audited by qualified technicians who can validate the deployment and ongoing PdM accuracy.
- Augment the existing solution with third-party tools. Pair the existing tech with higher-quality software or services from other vendors to bridge or augment gaps in PdM functionality or performance.
- Negotiate with the vendor. Contact the provider and express your displeasure. This can be used as leverage to secure additional support or receive discounted upgrades to help offset losses and improve the solution’s predictive maintenance accuracy in the long term.
Pitfall 3: Cybersecurity Vulnerabilities
According to a recent survey conducted by Fortinet, 73% of OT administrators reported they experienced intrusions that impacted OT systems in some way. This is up from only 49% in 2023. While the risk of cybersecurity often revolves around data theft or loss due to malware, another side effect of compromised OT and IoT systems is that predictive maintenance can also be negatively impacted. These compromised systems can either be inaccessible or impacted to where data collection becomes untrustworthy. This leads to skewed PdM predictions.
The good news is that despite OT and IoT having traditionally weak security compared to other parts of IT, the underlying network infrastructure that OT and IoT systems operate on can bolster protection. Here are a few examples:
- Network Segmentation. The IP network that smart building technologies use for data transport can be configured to strictly isolate OT and IoT systems from the rest of the network. This limits lateral movement by hackers if one device is compromised. Thus, if a single device or system becomes compromised, it cannot spread to other IoT devices or systems. This reduces the chance that devices will be unable to transmit smart building health and performance data, leading to improved predictive maintenance accuracy.
- Anomaly Detection. Network detection and response (NDR) tools use AI to monitor and baseline network traffic patterns. These systems can monitor OT/IoT systems and catch them when their behaviors veer, often indicating system or data compromise.
- Data Encryption. Data traversing a smart building network can be deleted or altered, skewing predictive maintenance processes. To protect the integrity of data as it moves from OT systems and IoT sensors to centralized data collection and analysis systems, be sure to enable end-to-end encryption. This prevents hackers from intercepting or altering data in transit.
- Automated Patch Management. In many cases, OT and IoT systems and hardware are vulnerable because the smart building IT staff has not yet applied the appropriate security patches to them. One way to ensure that smart building system software is patched consistently is to automate the process of applying and validating firmware updates.
Conclusion
Predictive maintenance accuracy often receives a bad reputation for the wrong reasons. Problems with poor data, substandard technology, and cybersecurity vulnerabilities can derail these systems, resulting in skewed predictions and operational challenges. The good news is that these issues can be resolved with some effort, leading to PdM recommendations and schedules that are both reliable and easy to maintain.
Next Steps for Building Owners and Smart Tech Integrators:
- Audit and calibrate your data sources regularly. Scheduling ongoing data quality checks and sensor recalibrations to ensure AI systems receive clean, consistent inputs is an important step facilities professionals don’t want to overlook.
- Invest in higher-quality or augmented tech. If you’re stuck with underperforming systems that produce inaccurate or insufficient data, it may be time to explore vendor tuning, third-party integrations, or negotiations for better support and upgrades.
- Strengthen cybersecurity across all endpoints. Robust cybersecurity measures are no longer an option for building professions—they’re a must. Work with your IT department to implement network segmentation, NDR tools, encrypted data transport, and automated patch management to protect PdM infrastructure from cyber threats.
- Reassess PdM frameworks quarterly. Schedule time for regular reviews of your predictive maintenance systems to ensure they align with operational goals and the latest technological advancements. Work with consultants or vendors if necessary to keep PdM frameworks operating at peak performance.
- Train staff on PdM limitations and best practices. Make sure your maintenance teams understand how data quality, tech calibration, and security practices impact PdM reliability. Remember: AI can only produce accurate outcomes when the data it analyzes and uses to make decisions is solid.
About the Author
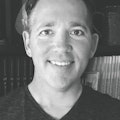
Andrew Froehlich
Contributor
As a highly regarded network architect and trusted IT consultant with worldwide contacts, Andrew Froehlich counts over two decades of experience and possesses multiple industry certifications in the field of enterprise networking. Andrew is the founder and president of Colorado-based West Gate Networks, which specializes in enterprise network architectures and data center build-outs. He’s also the founder of an enterprise IT research and analysis firm, InfraMomentum. As the author of two Cisco certification study guides published by Sybex, he is a regular contributor to multiple enterprise IT-related websites and trade journals with insights into rapidly changing developments in the IT industry.